Advantage of Pandas.
Practical Use of Pandas.
from pandas import DataFrame import matplotlib.pyplot as plt import numpy as np a=np.array([[4,8,5,7,6],[2,3,4,2,6],[4,7,4,7,8],[2,6,4,8,6],[2,4,3,3,2]]) df=DataFrame(a, columns=['a','b','c','d','e'], index=[2,4,6,8,10]) df.plot(kind='bar') # Turn on the grid plt.minorticks_on() plt.grid(which='major', linestyle='-', linewidth='0.5', color='green') plt.grid(which='minor', linestyle=':', linewidth='0.5', color='black') plt.show()
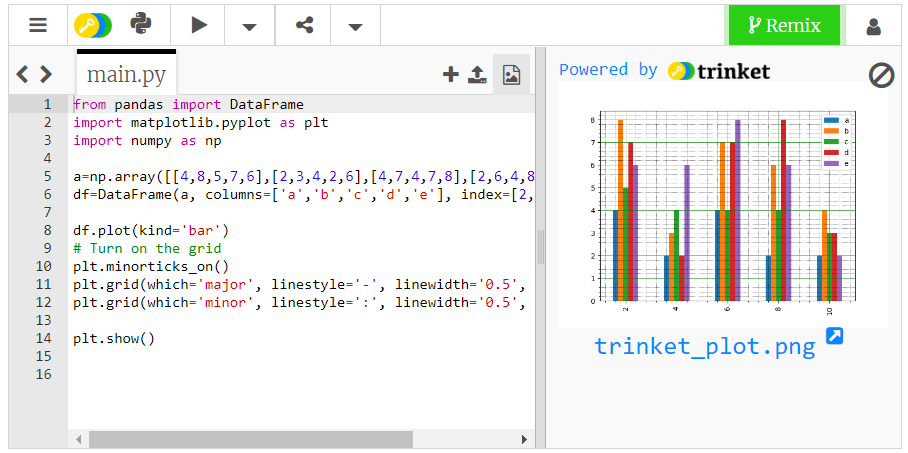
Commonly used Pandas methods.
Creating Data Frames/Series from scratch.
Pandas Data Frame can be created from a Python Dictionary. The keys in a dictionary becomes a column header and the array values for the key becomes the rows for that header.
import pandas as pd # declare a dataframe df = pd.DataFrame( { "Name": [ "Braund, Mr. Owen Harris", "Allen, Mr. William Henry", "Bonnell, Miss. Elizabeth", ], "Age": [22, 35, 58], "Sex": ["male", "male", "female"], } ) print(df)
On the other hand, Pandas Series can be created either from a Python Dictionary or derived from an existing Pandas Data Frame.
import pandas as pd # (1) declare a series from a dictionary Ages1 = pd.Series( { "Age": [22, 35, 58], } ) print(type(Ages1)) print(Ages1) print("----------------------------------------") # (2) declare a series from a previously declared dataframe Ages2 = df["Age"] print(type(Ages2)) print(Ages2)
Pandas Data Frame and Series can also be created from a Python List. However, the resulting dataframe/series will be missing the column names.
import pandas as pd # declare a dataframe df = pd.DataFrame( [ ["Braund, Mr. Owen Harris",22,"male"], ["Allen, Mr. William Henry",35,"male"], ["Bonnell, Miss. Elizabeth",58,"female"], ], ) print(df) print("----------------------------------------") # declare a series ds = pd.Series( [22, 35, 58], ) print(ds)
Alternatively, add an additional parameter (columns for dataframe and name for series) that defines the column names in the dataframe/series declaration statement.
import pandas as pd # declare a dataframe df = pd.DataFrame( [ ["Braund, Mr. Owen Harris",22,"male"], ["Allen, Mr. William Henry",35,"male"], ["Bonnell, Miss. Elizabeth",58,"female"], ], columns=["Name","Age","Sex"] ) print(df) print("----------------------------------------") # declare a series ds = pd.Series( [22, 35, 58], name="Name" ) print(ds)
Import/Export Data Frames/Series from/to external sources.
Usually the data source for Data Frame and Series are imported from text files e.g. CSV.
import pandas as pd # declare a dataframe from a remote file filepath="https://archive.org/download/misc-dataset/titanic.csv" df_titanic = pd.read_csv(filepath) print(type(df_titanic)) print(df_titanic[["PassengerId","Survived","Pclass"]])
Conversely, the Data Frame can be exported back to CSV file. Besides CSV, Data Frame can be exported to several other formats i.e. using methods to_dict(), to_excel(), to_json(), to_numpy() etc. (Read further: How do I read and write tabular data?).
import pandas as pd # declare a csv targetfilepath targetfilepathcsv="titanic.csv" df_titanic.to_csv(targetfilepathcsv) # declare a csv targetfilepath targetfilepathxls="titanic.xls" df_titanic.to_excel(targetfilepathxls)
Preview Data.
import pandas as pd # declare a dataframe from a remote file filepath="https://archive.org/download/misc-dataset/titanic.csv" df_titanic = pd.read_csv(filepath) print(df_titanic.head()) # the output shows head data(default 5 rows)
import pandas as pd # declare a dataframe from a remote file filepath="https://archive.org/download/misc-dataset/titanic.csv" df_titanic = pd.read_csv(filepath) print(df_titanic.tail()) # the output shows tail data(default 5 rows)
import pandas as pd # declare a dataframe from a remote file filepath="https://archive.org/download/misc-dataset/titanic.csv" df_titanic = pd.read_csv(filepath) print(df_titanic[500:505]) # the output shows a slice of data
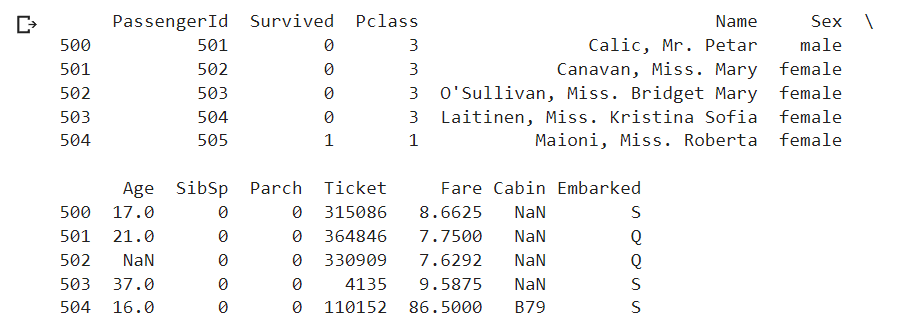
Preview Meta Data.
import pandas as pd # declare a dataframe from a remote file filepath="https://archive.org/download/misc-dataset/titanic.csv" df_titanic = pd.read_csv(filepath) print(df_titanic.info()) # the output shows: # df data type # range index # column description
Preview Data Description.
import pandas as pd # declare a dataframe from a remote file filepath="https://archive.org/download/misc-dataset/titanic.csv" df_titanic = pd.read_csv(filepath) print(df_titanic.shape) # the output shows: # (row size/column sizes)
import pandas as pd # declare a dataframe from a remote file filepath="https://archive.org/download/misc-dataset/titanic.csv" df_titanic = pd.read_csv(filepath) print(df_titanic.describe()) # the output shows: # descriptive statistics of the data
Selecting Specific Row/Column of Data.
import pandas as pd # declare a dataframe from a remote file filepath="https://archive.org/download/misc-dataset/titanic.csv" df_titanic = pd.read_csv(filepath) # using loc i.e. select by label location print(df_titanic.loc[df_titanic['Age'].isna()==False,['Age']])
import pandas as pd # declare a dataframe from a remote file filepath="https://archive.org/download/misc-dataset/titanic.csv" df_titanic = pd.read_csv(filepath) # using iloc i.e. select by integer location print(df_titanic.iloc[list(df_titanic.Age.isna()==False),[5]])
Dropping Data.
import pandas as pd # declare a dataframe from a remote file filepath="https://archive.org/download/misc-dataset/titanic.csv" df_titanic = pd.read_csv(filepath) df_titanic = df_titanic.drop(columns=['SibSp', 'Parch', 'Ticket', 'Fare','Cabin','Embarked']) print(df_titanic.info()) # the output shows: # remaining 6 columns # (the other 6 columns have been dropped)
import pandas as pd # declare a dataframe from a remote file filepath="https://archive.org/download/misc-dataset/titanic.csv" df_titanic = pd.read_csv(filepath) df_titanic = df_titanic.dropna() print(df_titanic.info()) # the output shows: # remaining 183 rows # (other 714 rows with missing values have been dropped)
Imputing Data.
import pandas as pd # declare a dataframe from a remote file filepath="https://archive.org/download/misc-dataset/titanic.csv" df_titanic = pd.read_csv(filepath) print(df_titanic.info()) df_titanic.fillna(0, inplace = True) print(df_titanic.info())
import pandas as pd # declare a dataframe from a remote file filepath="https://archive.org/download/misc-dataset/titanic.csv" df_titanic = pd.read_csv(filepath) age_mean=int(df_titanic['Age'].mean()) print(age_mean) # replacing missing values with mean value df_titanic.fillna(age_mean, inplace = True) # select the first 10 rows and column Age print(df_titanic.loc[:10,['Age']])
0 Comments